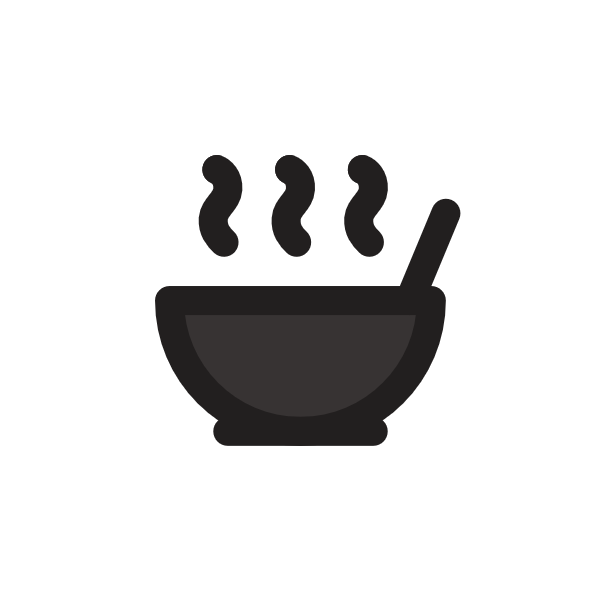
Cocojunk
🚀 Dive deep with CocoJunk – your destination for detailed, well-researched articles across science, technology, culture, and more. Explore knowledge that matters, explained in plain English.
Survivorship bias
Read the original article here.
Understanding Survivorship Bias: How Filtered Data Shapes Perception and Can Lead to Manipulation
In the digital age, we are constantly bombarded with data – news feeds, search results, social media posts, performance metrics, advertising, and success stories. However, the data we see is often only a fraction of the total data that exists. Much is filtered, curated, or simply disappears from view. This selective presentation of information can lead to a powerful cognitive bias known as survivorship bias.
This resource will delve into the concept of survivorship bias, exploring its definition, common manifestations, and how it plays a crucial role in shaping our understanding, often without our conscious awareness. Understanding this bias is particularly vital in the context of "Digital Manipulation," as the platforms and algorithms that curate our digital experiences are prime architects of data filtering.
What is Survivorship Bias?
Survivorship Bias (or Survival Bias): A logical error and a form of sampling bias where one concentrates only on the data points, entities, or information that survived some selection process, while completely overlooking or failing to account for those that did not.
This bias leads to incorrect conclusions because the analysis is based on incomplete and unrepresentative data – specifically, the data from failures, dropouts, or those filtered out is missing. By only looking at the "survivors," we can develop overly optimistic views, attribute success to incorrect factors, or miss critical insights hidden in the data of those who didn't make it through the selection process.
Think of it as looking at a group of highly successful people and trying to find common traits that caused their success, without also looking at the countless others who had similar traits but failed. You only see the successful outcome, not the many unsuccessful ones that were filtered out.
Survivorship Bias as a General Experimental Flaw
Survivorship bias is not limited to specific fields; it's a fundamental flaw that can impact any analysis where a selection process has occurred, and only the results of the "survivors" are considered.
Example: Early Parapsychology Research
- The Scenario: Researcher Joseph Banks Rhine studied Extra-Sensory Perception (ESP) using Zener cards. He identified a few subjects who consistently guessed the cards with statistically improbable accuracy, leading him to believe they possessed ESP.
- The Bias: Critics argued that Rhine overlooked the hundreds or thousands of subjects he tested who failed to show any ESP ability. He focused only on the few "successful" subjects (the survivors of his testing process).
- The Explanation: If you test a large enough group, pure chance dictates that a few individuals will achieve seemingly improbable success just through random luck. By only looking at these lucky few and ignoring the vast majority who failed, Rhine was subject to survivorship bias. He saw their results in isolation, rather than as outliers in a much larger, less successful pool.
Survivorship Bias in Research Publication
This concept extends to scientific research itself, especially before safeguards were widely adopted.
- The Scenario: Imagine many scientists are researching the same phenomenon. Some experiments will yield statistically significant results purely by chance, even if the phenomenon isn't real (false positives).
- The Bias: Experiments that find positive, statistically significant results are more likely to be submitted and accepted for publication ("Positive Results Bias" or "Publication Bias"). Experiments that fail to find a significant result (confirming the "null hypothesis" - essentially showing nothing happened) are less likely to be published.
- The Outcome: Readers of scientific literature primarily see the "surviving" studies – those with positive results. This creates a distorted view, making the phenomenon seem more robust or real than it might be if all the failed studies were also considered. This bias contributes to the difficulty in replicating many published research findings.
Positive Results Bias / Publication Bias: The tendency for studies with statistically significant or positive results to be published more frequently than studies with non-significant or negative results, leading to a biased view of the overall evidence.
Example: The "Immortal Time Bias" in Medical Studies
- The Scenario: A study claimed that Academy Award-winning actors and actresses lived significantly longer than their less successful peers.
- The Bias: The statistical method included all of a winner's years of life before they won the award in the calculation of their survival after winning. Winning is a selection event that happens later in life. By counting the years lived up to winning as "survival after winning," the study gave winners an unfair advantage.
- The Outcome: This is a form of "Immortal Time Bias" because the time before the event (winning) is included as time after the event. When reanalyzed correctly, the survival advantage was much smaller and not statistically significant. The initial analysis essentially included a period where the "survivors" (winners) were guaranteed to be alive because they hadn't won yet, distorting the comparison.
Immortal Time Bias: A form of survivorship bias specific to time-based studies, where a period of follow-up during which an outcome event cannot occur (because the event defines the start of follow-up) is incorrectly included in the calculation of survival time, unfairly favoring the "exposed" or "surviving" group.
Examples of Survivorship Bias in Various Fields
Survivorship bias appears in many domains, affecting our understanding of everything from finance to history and even animal behavior.
1. Finance and Economics
- The Scenario: Analyzing the performance of mutual funds or companies over time.
- The Bias: Failed companies are excluded from analyses because they no longer exist. Mutual funds that perform poorly are often closed or merged into other funds. Performance studies frequently only include funds or companies that survive until the end of the study period.
- The Outcome: The results of these studies are skewed upwards. If you only look at the currently existing funds and their past performance, you miss the data from the many funds that failed, making average performance seem much higher than it actually was for all funds that started. Similarly, analyzing a stock index like the S&P 500 requires using the actual historical index members, not just the current ones, because companies are added and removed based on performance; using only current members biases historical performance upwards.
Alpha (α) in Finance: A measure used to gauge the performance of an investment (like a mutual fund) relative to a benchmark index (like the S&P 500), while accounting for risk. A positive alpha indicates outperformance, while a negative alpha indicates underperformance. Survivorship bias inflates calculated average alpha because poorly performing funds with negative alpha are excluded.
2. Business and Entrepreneurship
- The Scenario: Giving or receiving advice about how to succeed in business, especially from highly successful entrepreneurs.
- The Bias: We tend to hear the stories and advice only from those who made it (the survivors: Mark Zuckerberg, Bill Gates, etc.). We rarely hear from the thousands or millions of people who had similar backgrounds, ideas, or work ethics but whose businesses failed.
- The Outcome: This creates a distorted picture of success. It makes success seem easier, more dependent on specific traits of the individual, or less subject to luck or external factors than it is. The vast "silent evidence" of failure is ignored. Advice from survivors might highlight factors they believe were crucial, but these might not be the true determinants of success, or they might be factors shared by many who failed.
3. History
- The Scenario: Historians studying organizations or events.
- The Bias: Organizations that survive for a long time (like long-standing charities or institutions) are more likely to have preserved archives and accessible records. Organizations that failed or were short-lived are less likely to have left behind easily accessible documentation.
- The Outcome: Historians may disproportionately study the long-standing, successful organizations because the data (archives) from the "non-survivors" is missing or difficult to find. This can lead to an incomplete or biased understanding of a historical period or type of organization.
4. Highly Competitive Careers
- The Scenario: Observing success in fields like acting, music, professional sports, or highly sought-after corporate roles.
- The Bias: We see the movie stars, famous athletes, chart-topping musicians, and high-flying CEOs (the survivors). We don't see the countless individuals who were equally talented, dedicated, or educated but never achieved significant success due to luck, timing, connections, or factors beyond their control (the vast majority who didn't survive the intense competition).
- The Outcome: This creates unrealistic expectations about the likelihood of achieving such success and reinforces the myth that talent and hard work alone guarantee reaching the top. It ignores the enormous number of failures that are invisible to the public eye. Online platforms exacerbate this by highlighting viral successes while the millions of creators who never gain traction remain unseen.
5. Military Strategy (A Famous Example)
- The Scenario: During World War II, statistician Abraham Wald was asked by the U.S. military where to add armor to bombers to minimize losses. They examined returning planes and noted where the bullet holes were concentrated.
- The Initial Thought (Subject to Bias): Add armor to the areas with the most bullet holes, assuming these areas are frequently hit.
- Wald's Analysis (Avoiding Bias): Wald realized the data (bullet holes on returning planes) was subject to survivorship bias. The returning planes were the survivors. The bullet holes showed where planes could be hit and still survive the mission. The planes that didn't return must have been hit in areas where the returning planes showed no damage – because hits in those areas were fatal.
- The Outcome: Wald recommended adding armor to the areas that showed the least damage on the returning planes, correctly inferring that these were the critical areas where hits resulted in the aircraft being lost. This classic example perfectly illustrates the danger of only analyzing the survivors.
6. Animal Behavior
- The Scenario: A study on cats falling from buildings noted that cats falling from higher stories seemed to have fewer injuries than cats falling from lower stories (6 stories or less).
- The Bias: One proposed explanation (besides the biological one about terminal velocity and relaxation) is survivorship bias. Cats that fall from very high distances and die are less likely to be taken to a veterinarian and thus wouldn't be included in a study based on vet records. The study only captured the data of the surviving, injured cats brought to the vet. Cats that fell from lower heights were more likely to survive any fall, injured or not, and thus a higher proportion of injured (but surviving) cats from lower falls might end up in the vet data compared to high falls where death was the more likely outcome.
- The Outcome: The data set (cats brought to the vet) was inherently biased towards survivors, potentially skewing the perceived relationship between fall height and injury severity.
7. Business Law and Advertising (An Explicit Case of Data Filtering)
- The Scenario: Online services advertising high success rates (e.g., dating sites, job placement services).
- The Bias: The company might deliberately pre-screen potential customers, only accepting those with characteristics that make them more likely to succeed with the service. They then calculate their success rate based only on this pre-selected group (the survivors of their screening process), while advertising this rate to the general public, many of whom would not pass the initial screening.
- The Outcome: The advertised success rate is misleading because the target audience includes people who would be filtered out. The company is using data from a biased sample (their accepted clients) to make claims about potential outcomes for a broader, un-screened population. This is a deliberate use of data filtering to manipulate perceived success rates.
Connecting Survivorship Bias to Digital Manipulation: How Data Filtering Shapes Our Reality
The core mechanism of survivorship bias – filtering out failures or non-selected entities – is deeply embedded in how digital platforms operate and present information. This makes understanding the bias crucial for recognizing potential digital manipulation.
1. Algorithms and Filter Bubbles:
- The Mechanism: Social media feeds, news aggregators, and search engines use complex algorithms to decide what content you see. They prioritize content based on engagement, personalization, potential virality, or other factors. Content that doesn't meet these criteria is filtered out or ranked lower, effectively becoming a "non-survivor" in your feed.
- The Bias: You are constantly shown the "survivors" of the algorithm's selection process. This creates filter bubbles or echo chambers, where you are primarily exposed to information, opinions, or people that align with your existing views or are deemed most likely to keep you engaged.
- The Manipulation: This isn't necessarily malicious, but it is a form of shaping your reality. By only showing you certain viewpoints, products, or news stories, platforms can influence your opinions, consumption habits, and understanding of the world. You lose sight of the vast amount of information that didn't "survive" the algorithm's filter.
2. Online Reviews and Ratings:
- The Mechanism: Many businesses allow customers to leave reviews. However, companies might use tactics like removing negative reviews that violate loosely defined terms, only soliciting reviews from satisfied customers, or making it harder to leave low ratings.
- The Bias: The collection of visible reviews becomes biased towards positive outcomes (the "survivors" of the review filtering process).
- The Manipulation: Potential customers see an artificially inflated positive sentiment, leading them to make purchasing decisions based on incomplete and misleading data.
3. Curated Online Success Stories:
- The Mechanism: Social media, online courses, and marketing materials are filled with stories of viral success – the influencer who gained millions of followers overnight, the startup that became a unicorn, the person who got rich trading crypto.
- The Bias: You primarily see and hear about the "survivors" of intense competition or highly risky ventures. The millions of people who tried and failed are invisible.
- The Manipulation: This fuels unrealistic aspirations and can lead people to invest time, money, or effort into paths that are statistically highly unlikely to succeed, based on a biased sample of visible outcomes. Online marketers often leverage this by showcasing only successful testimonials.
4. Data Analysis in Digital Marketing and Politics:
- The Mechanism: Digital campaigns analyze data to optimize targeting, messaging, and strategy. They might focus on metrics like click-through rates or conversion rates among users who engaged with the content.
- The Bias: Analysts might overlook the data from the vast majority of users who didn't click, convert, or engage. Focusing only on the "survivors" of the user journey can lead to a skewed understanding of campaign effectiveness or user behavior.
- The Manipulation: This biased analysis can lead to flawed strategies, reinforcing tactics that appear successful within the filtered data, but fail to address the reasons why a large proportion of the audience was unaffected or alienated. In political campaigns, focusing only on data from likely supporters can lead to neglecting or misunderstanding the concerns of other groups.
5. Personalized Advertising:
- The Mechanism: Advertisers use data to target specific user segments deemed most likely to be interested or convert.
- The Bias: While efficient for targeting, this means data on why the vast majority of people aren't interested or don't convert is less emphasized in successful campaign reports. The focus is on the "survivors" of the targeting and conversion funnel.
- The Manipulation: Users are shown products or services they are predisposed to engage with. While this can be helpful, it also limits exposure to alternatives and reinforces existing preferences, potentially narrowing consumer choices or influencing desires based on a filtered view of what's available or desirable.
The Consequences in the Digital Age
In a world where much of our information and interaction is mediated by digital platforms, survivorship bias has significant consequences:
- Distorted Reality: Our understanding of the world, the likelihood of success, the prevalence of opinions, and the quality of products can be fundamentally skewed by only seeing filtered data.
- Unrealistic Expectations: Seeing only the highlight reels of success online fosters a belief that such outcomes are common or easily achievable.
- Increased Susceptibility to Manipulation: When we don't question what isn't shown, we are more easily influenced by the curated narrative presented by platforms, marketers, or political actors.
- Reduced Critical Thinking: If we aren't aware of the filtering, we are less likely to seek out alternative perspectives or look for data on failures.
How to Navigate Filtered Data and Mitigate Survivorship Bias
Becoming aware of survivorship bias is the first step. In the digital realm, this means actively questioning the data and information presented to you:
- Ask: "What Am I Not Seeing?": Whenever presented with success stories, positive reviews, or curated feeds, consider the missing data. Who failed? What negative experiences aren't being shown? What information was filtered out?
- Seek Diverse Sources: Actively look for information from multiple perspectives and platforms, including those that might challenge your existing views.
- Look for Data on Failures: In analyses, ask about churn rates, dropout rates, unsuccessful attempts, or negative outcomes, not just successes.
- Be Critical of Anecdotes: Understand that personal success stories, while inspiring, are often the "survivors" and not representative of the average outcome.
- Understand Platform Algorithms: Learn how the platforms you use curate content. While you can't control the algorithm, understanding its purpose helps you recognize the filtering process.
By recognizing that the data we encounter, especially online, is often the result of complex selection processes, we can approach information with a more critical eye and make more informed judgments, reducing the risk of being manipulated by incomplete or biased narratives. Survivorship bias reminds us that sometimes, the most important data is the data that isn't there.
Related Articles
See Also
- "Amazon codewhisperer chat history missing"
- "Amazon codewhisperer keeps freezing mid-response"
- "Amazon codewhisperer keeps logging me out"
- "Amazon codewhisperer not generating code properly"
- "Amazon codewhisperer not loading past responses"
- "Amazon codewhisperer not responding"
- "Amazon codewhisperer not writing full answers"
- "Amazon codewhisperer outputs blank response"
- "Amazon codewhisperer vs amazon codewhisperer comparison"
- "Are ai apps safe"